Introduction
In our earlier blog post A Simpler Alternative to Kubernetes – Docker Swarm with Swarmpit we talked about how Docker Swarm, a popular open-source orchestration platform along with Swarmpit, another open-source simple and intuitive Swarm Monitoring and Management Web User interface for Swarm, we can do most of the activities that one does with Kubernetes, including the deployment and management of containerized applications across a cluster of Docker hosts with clustering and load balancing enabling for simple applications.
We know monitoring container logs efficiently is crucial for troubleshooting and maintaining application health in a Docker environment. In this article, we explore how this can be achieved easily using Swarmpit’s features for real-time monitoring of service logs with its strong filtering capabilities, and best practices for log management.
Challenges in Log Monitoring
Managing logs in a distributed environment like Docker Swarm presents several challenges:
- Logs are distributed across multiple containers and nodes.
- Accessing logs requires logging into individual nodes or using CLI tools.
- Troubleshooting can be time-consuming without a centralized interface.
Swarmpit's Logging Features
Swarmpit simplifies log monitoring by providing a centralized UI for viewing container logs without accessing nodes manually.
Key Capabilities:
- Real-Time Log Viewing: Monitor logs of running containers in real-time.
- Filtering and Searching: Narrow down logs using service names, container IDs, or keywords.
- Replica-Specific Logs: View logs for each replica in a multi-replica service.
- Log Retention: Swarmpit retains logs for a configurable period based on system resources.
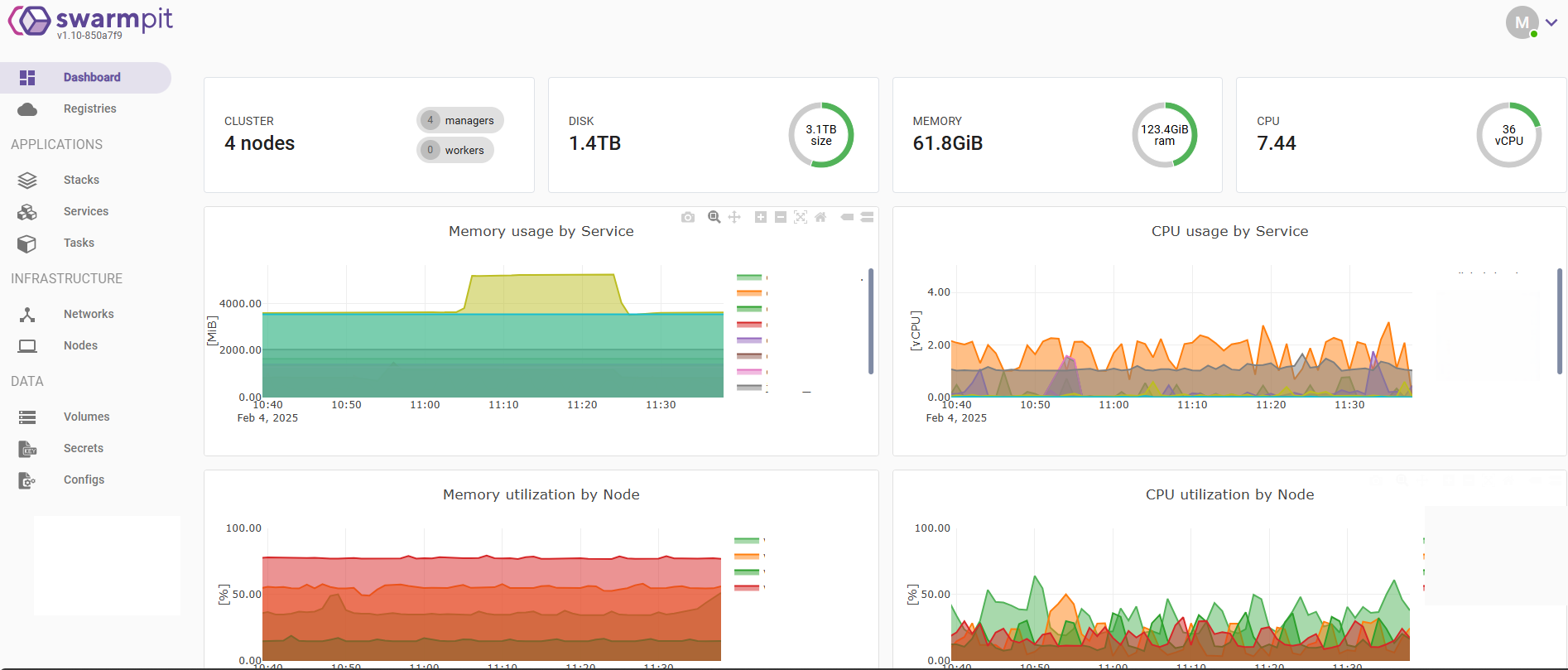
Accessing Logs in Swarmpit
To access logs in Swarmpit:
- Log in to the Swarmpit dashboard.
- Navigate to the Services or Containers tab.
- Select the service or container whose logs you need.
- Switch to the Logs tab to view real-time output.
- Use the search bar to filter logs by keywords or service names.
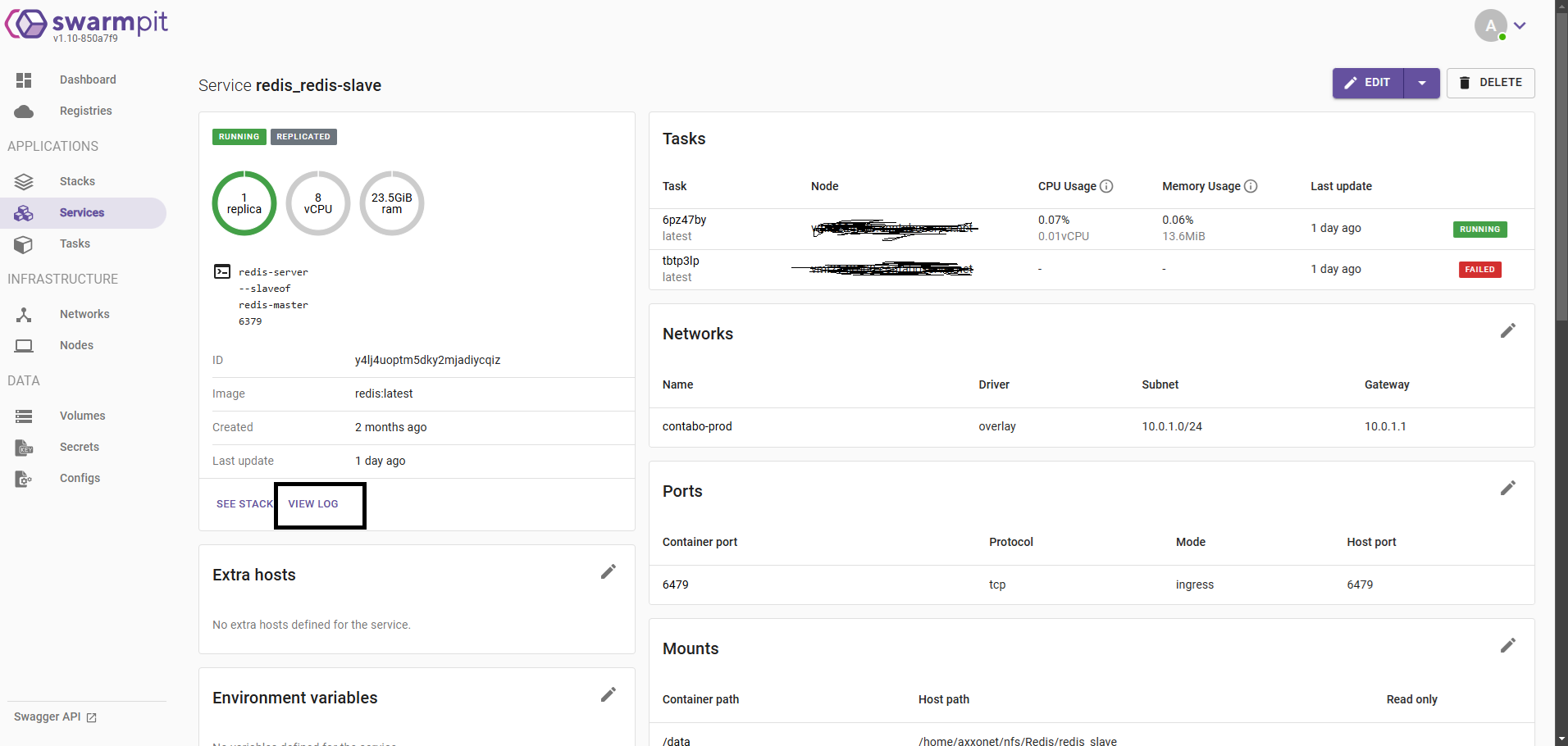
Filtering and Searching Logs
Swarmpit provides advanced filtering options to help you locate relevant log entries quickly.
- Filter by Service or Container: Choose a specific service or container from the UI.
- Filter by Replica: If a service has multiple replicas, select a specific replica’s logs.
- Keyword Search: Enter keywords to find specific log messages.
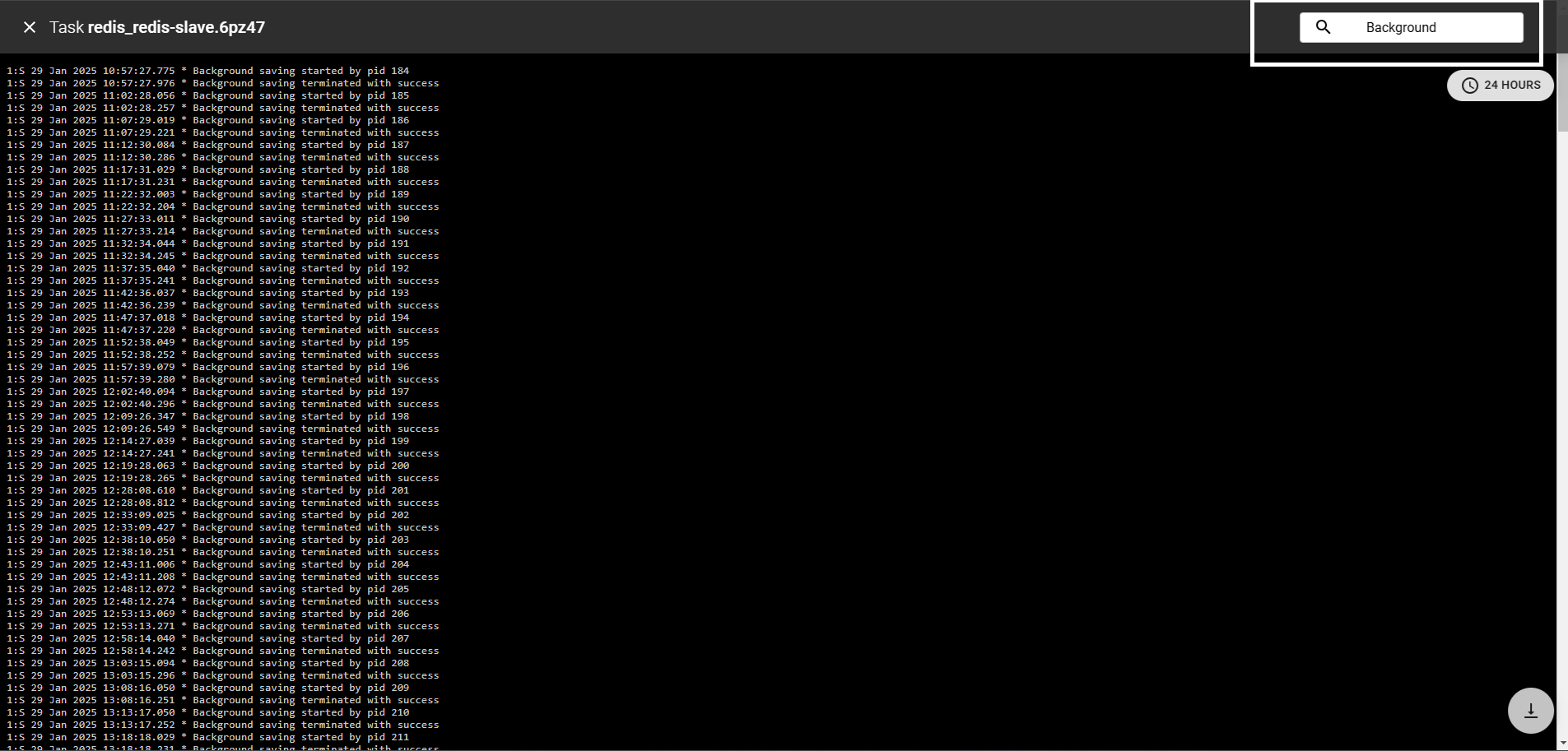
Best Practices for Log Monitoring
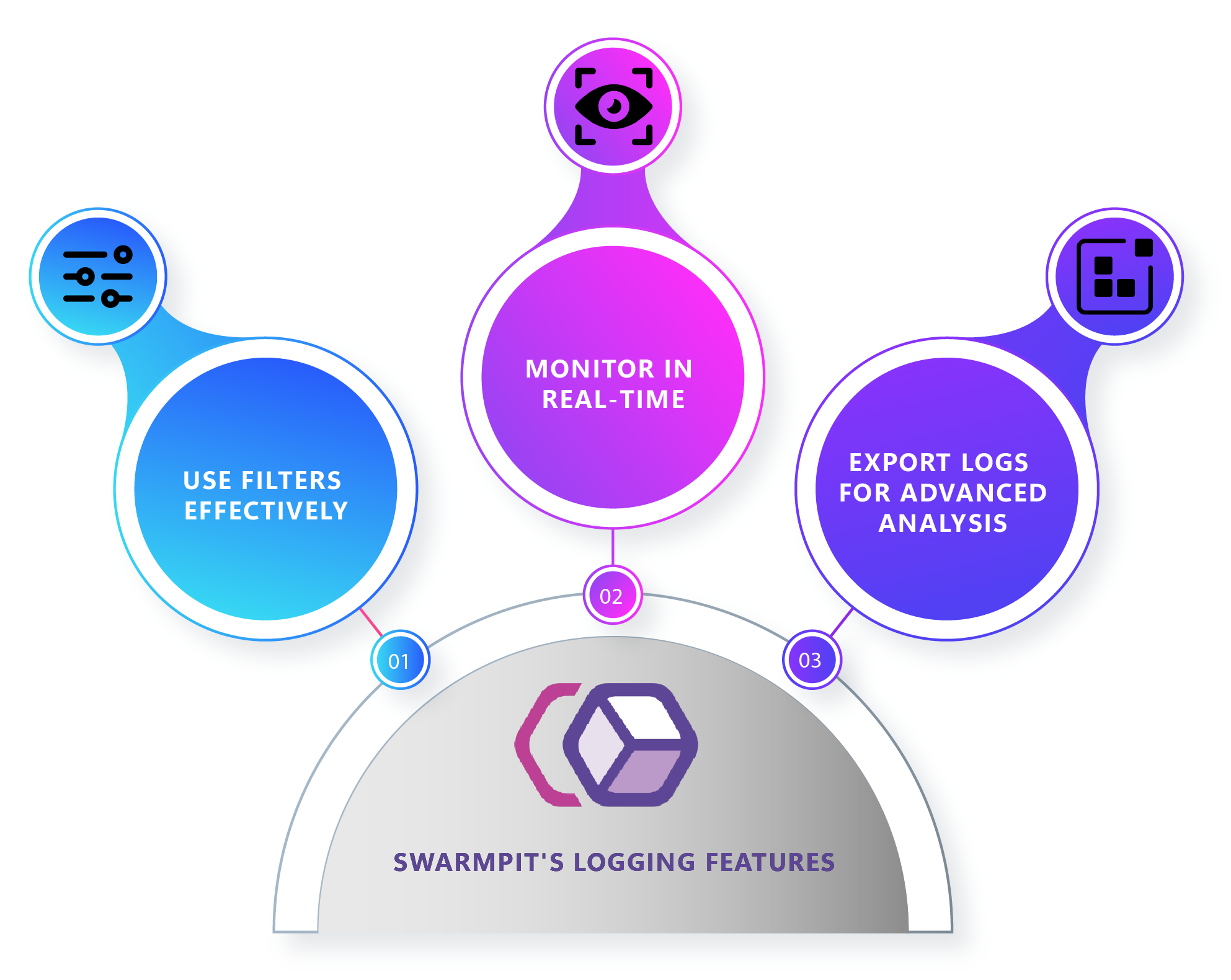
To make the most of Swarmpit’s logging features:
- Use Filters Effectively: Apply filters to isolate logs related to specific issues.
- Monitor in Real-Time: Keep an eye on live logs to detect errors early.
- Export Logs for Advanced Analysis: If deeper log analysis is required, consider integrating with external tools like Loki or ELK.
Conclusion
Swarmpit’s built-in logging functionality provides a simple and effective way to monitor logs in a Docker Swarm cluster. By leveraging its real-time log viewing and filtering capabilities, administrators can troubleshoot issues efficiently without relying on additional logging setups. While Swarmpit does not provide node-level logs, its service, and replica-specific log views offer a practical solution for centralized log monitoring.
This guide demonstrates how to utilize Swarmpit’s logging features for streamlined log management in a Swarm cluster.
Optimize your log management with the right tools. Get in touch to learn more!
Frequently Asked Questions (FAQs)
No, Swarmpit provides logs per service and per replica but does not offer node-level log aggregation.
Log retention depends on system resources and configuration settings within Swarmpit.
Yes, for advanced log analysis, you can integrate Swarmpit with tools like Loki or the ELK stack.
No, Swarmpit does not provide built-in log alerting. For alerts, consider integrating with third-party monitoring tools.
Yes, Swarmpit allows keyword-based filtering, enabling you to search for specific error messages.