Introduction
Apache Druid is a distributed, column-oriented, real-time analytics database designed for fast, scalable, and interactive analytics on large datasets. It excels in use cases requiring real-time data ingestion, high-performance queries, and low-latency analytics.
Druid was originally developed to power interactive data applications at Metamarkets and has since become a widely adopted open-source solution for real-time analytics, particularly in industries such as ad tech, fintech, and IoT.
It supports batch and real-time data ingestion, enabling users to perform fast ad-hoc queries, power dashboards, and interactive data exploration.
In big data and real-time analytics, having the right tools to process and analyze large volumes of data swiftly is essential. Apache Druid, an open-source, high-performance, column-oriented distributed data store, has emerged as a leading solution for real-time analytics and OLAP (online analytical processing) workloads. In this blog post, we’ll delve into what Apache Druid is, its key features, and how it can revolutionize your data analytics capabilities. Refer to the official documentation for more information.
Apache Druid
Apache Druid is a high-performance, real-time analytics database designed for fast slice-and-dice analytics on large datasets. It was created by Metamarkets (now part of Snap Inc.) and is now an Apache Software Foundation project. Druid is built to handle both batch and streaming data, making it ideal for use cases that require real-time insights and low-latency queries.
Key Features of Apache Druid:
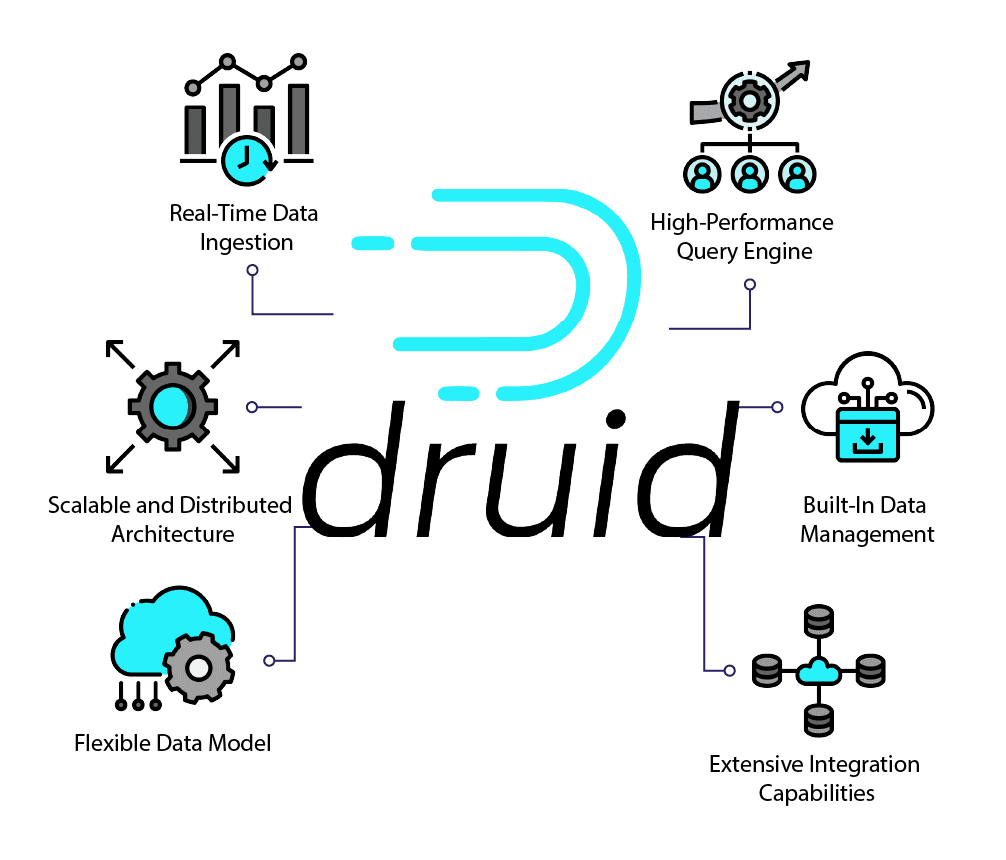
Real-Time Data Ingestion
Druid excels at real-time data ingestion, allowing data to be ingested from various sources such as Kafka, Kinesis, and traditional batch files. It supports real-time indexing, enabling immediate query capabilities on incoming data with low latency.
High-Performance Query Engine
Druid’s query engine is optimized for fast, interactive querying. It supports a wide range of query types, including Time-series, TopN, GroupBy, and search queries. Druid’s columnar storage format and advanced indexing techniques, such as bitmap indexes and compressed column stores, ensure that queries are executed efficiently.
Scalable and Distributed Architecture
Druid’s architecture is designed to scale horizontally. It can be deployed on a cluster of commodity hardware, with data distributed across multiple nodes to ensure high availability and fault tolerance. This scalability makes Druid suitable for handling large datasets and high query loads.
Flexible Data Model
Druid’s flexible data model allows for the ingestion of semi-structured and structured data. It supports schema-on-read, enabling dynamic column discovery and flexibility in handling varying data formats. This flexibility simplifies the integration of new data sources and evolving data schemas.
Built-In Data Management
Druid includes built-in features for data management, such as automatic data partitioning, data retention policies, and compaction tasks. These features help maintain optimal query performance and storage efficiency as data volumes grow.
Extensive Integration Capabilities
Druid integrates seamlessly with various data ingestion and processing frameworks, including Apache Kafka, Apache Storm, and Apache Flink. It also supports integration with visualization tools like Apache Superset, Tableau, and Grafana, enabling users to build comprehensive analytics solutions.
Use Cases of Apache Druid
Real-Time Analytics
Druid is used in real-time analytics applications where the ability to ingest and query data in near real-time is critical. This includes monitoring applications, fraud detection, and customer behavior tracking.
Ad-Tech and Marketing Analytics
Druid’s ability to handle high-throughput data ingestion and fast queries makes it a popular choice in the ad tech and marketing industries. It can track user events, clicks, impressions, and conversion rates in real time to optimize campaigns.
IoT Data and Sensor Analytics
IoT applications produce time-series data at high volume. Druid’s architecture is optimized for time-series data analysis, making it ideal for analyzing IoT sensor data, device telemetry, and real-time event tracking.
Operational Dashboards
Druid is often used to power operational dashboards that provide insights into infrastructure, systems, or applications. The low-latency query capabilities ensure that dashboards reflect real-time data without delay.
Clickstream Analysis
Organizations leverage Druid to analyze user clickstream data on websites and applications, allowing for in-depth analysis of user interactions, preferences, and behaviors in real time.
The Architecture of Apache Druid
Apache Druid follows a distributed, microservice-based architecture. The architecture allows for scaling different components based on the system’s needs.
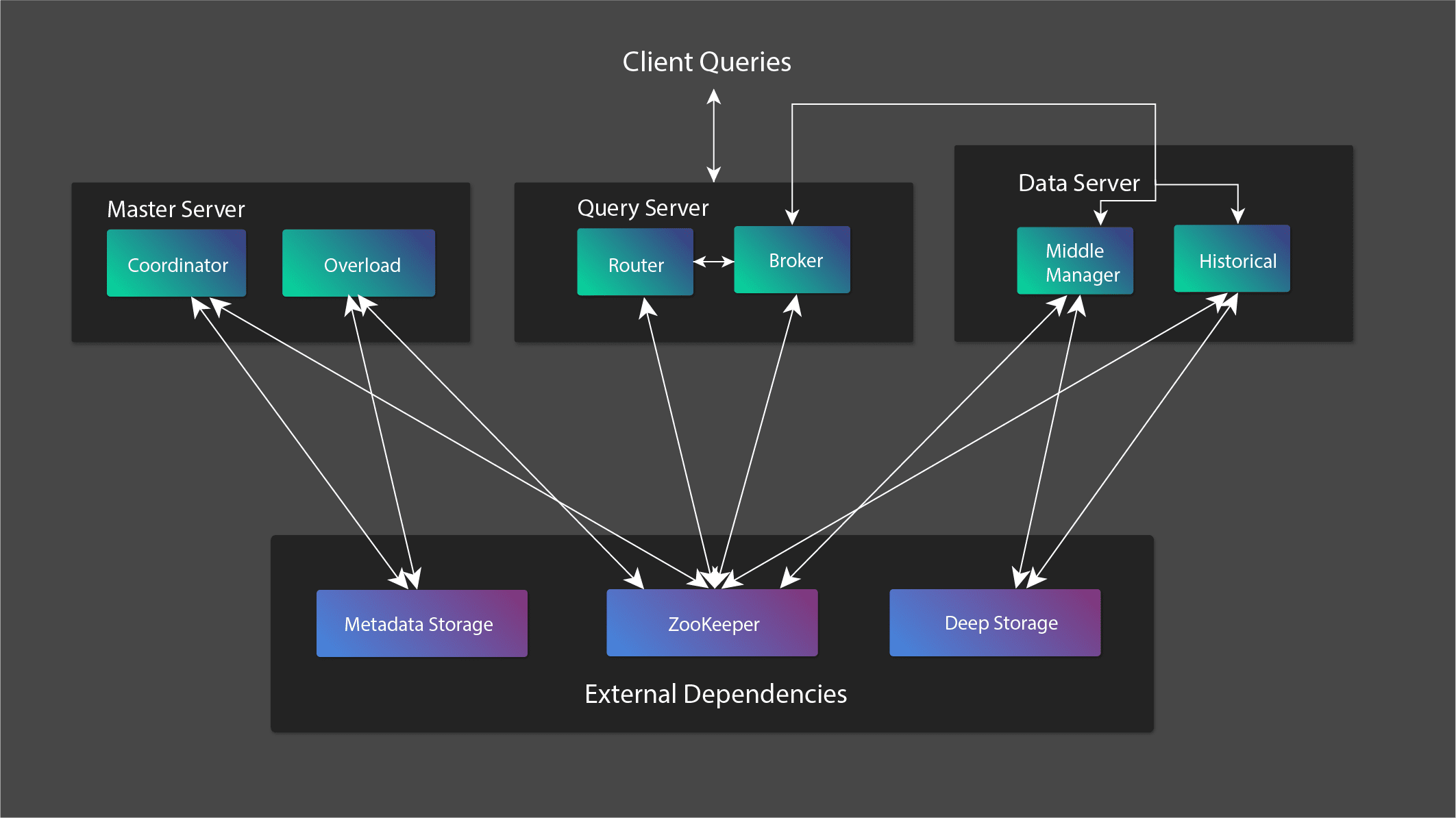
The main components are:
Coordinator and Overlord Nodes
- Coordinator Node: Manages data availability, balancing the distribution of data across the cluster, and overseeing segment management (segments are the basic units of storage in Druid).
- Overlord Node: Responsible for managing ingestion tasks. It works with the middle managers to schedule and execute data ingestion tasks, ensuring that data is ingested properly into the system.
Historical Nodes
Historical nodes store immutable segments of historical data. When queries are executed, historical nodes serve data from the disk, which allows for low-latency and high-throughput queries.
MiddleManager Nodes
MiddleManager nodes handle real-time ingestion tasks. They manage tasks such as ingesting data from real-time streams (like Kafka), transforming it, and pushing the processed data to historical nodes after it has persisted.
Broker Nodes
The broker nodes route incoming queries to the appropriate historical or real-time nodes and aggregate the results. They act as the query routers and perform query federation across the Druid cluster.
Query Nodes
Query nodes are responsible for receiving, routing, and processing queries. They can handle a variety of query types, including SQL, and route these queries to other nodes for execution.
Deep Storage
Druid relies on an external deep storage system (such as Amazon S3, Google Cloud Storage, or HDFS) to store segments of data permanently. The historical nodes pull these segments from deep storage when they need to serve data.
Metadata Storage
Druid uses an external relational database (typically PostgreSQL or MySQL) to store metadata about the data, including segment information, task states, and configuration settings.
Advantages of Apache Druid
- Sub-Second Query Latency: Optimized for high-speed data queries, making it perfect for real-time dashboards.
- Scalability: Easily scales to handle petabytes of data.
- Flexible Data Ingestion: Supports both batch and real-time data ingestion from multiple sources like Kafka, HDFS, and Amazon S3.
- Column-Oriented Storage: Efficient data storage with high compression ratios and fast retrieval of specific columns.
- SQL Support: Familiar SQL-like querying capabilities for easy data analysis.
- High Availability: Fault-tolerant and highly available due to data replication across nodes.
Getting Started with Apache Druid
Installation and Setup
Setting up Apache Druid involves configuring a cluster with different node types, each responsible for specific tasks:
- Master Nodes: Oversee coordination, metadata management, and data distribution.
- Data Nodes: Handle data storage, ingestion, and querying.
- Query Nodes: Manage query routing and processing.
You can install Druid using a package manager, Docker, or by downloading and extracting the binary distribution. Here’s a brief overview of setting up Druid using Docker:
- Download the Docker Compose File:
$curl -O https://raw.githubusercontent.com/apache/druid/master/examples/docker-compose/docker-compose.yml - Start the Druid Cluster: $ docker-compose up
- Access the Druid Console: Open your web browser and navigate to http://localhost:8888 to access the Druid console.
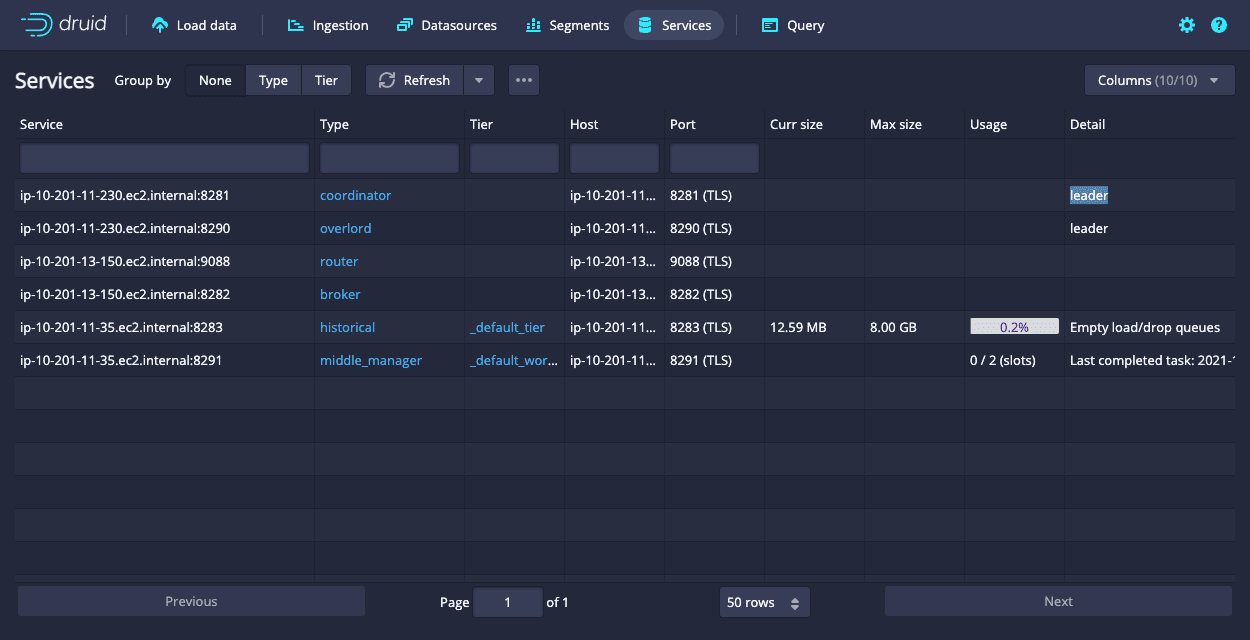
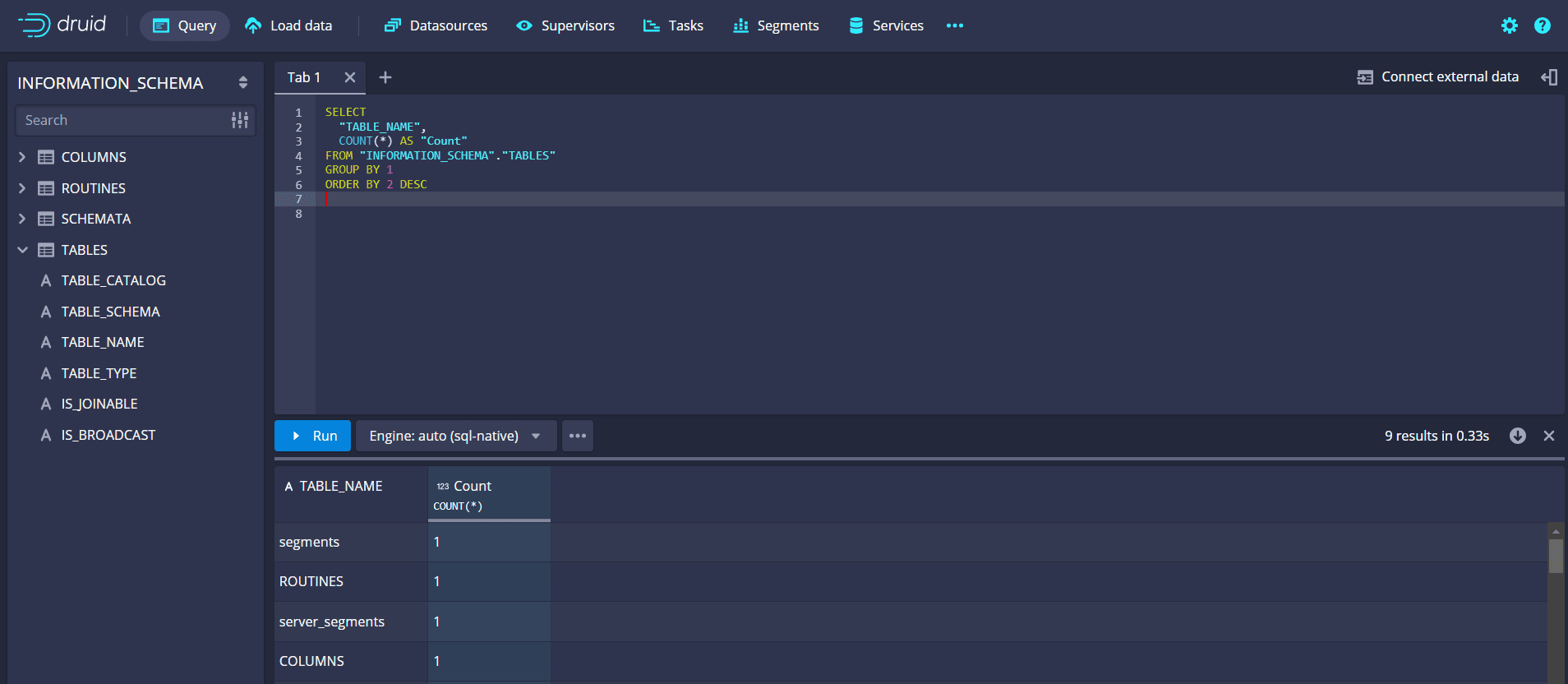
Ingesting Data
To ingest data into Druid, you need to define an ingestion spec that outlines the data source, input format, and parsing rules. Here’s an example of a simple ingestion spec for a CSV file:
JSON Code
{ “type”: “index_parallel”, “spec”: { “ioConfig”: { “type”: “index_parallel”, “inputSource”: { “type”: “local”, “baseDir”: “/path/to/csv”, “filter”: “*.csv” }, “inputFormat”: { “type”: “csv”, “findColumnsFromHeader”: true } }, “dataSchema”: { “dataSource”: “example_data”, “timestampSpec”: { “column”: “timestamp”, “format”: “iso” }, “dimensionsSpec”: { “dimensions”: [“column1”, “column2”, “column3”] } }, “tuningConfig”: { “type”: “index_parallel” } }} |
Submit the ingestion spec through the Druid console or via the Druid API to start the data ingestion process.
Querying Data
Once your data is ingested, you can query it using Druid’s native query language or SQL. Here’s an example of a simple SQL query to retrieve data from the example_data data source:
SELECT __time, column1, column2, columnFROM example_dataWHERE __time BETWEEN ‘2023-01-01’ AND ‘2023-01-31’ |
Use the Druid console or connect to Druid from your preferred BI tool to execute queries and visualize data.
Conclusion
Apache Druid is a powerful, high-performance real-time analytics database that excels at handling large-scale data ingestion and querying. Its robust architecture, flexible data model, and extensive integration capabilities make it a versatile solution for a wide range of analytics use cases. Whether you need real-time insights, interactive queries, or scalable OLAP capabilities, Apache Druid provides the tools to unlock the full potential of your data. Explore Apache Druid today and transform your data analytics landscape. Apache Druid has firmly established itself as a leading database for real-time, high-performance analytics. Its unique combination of real-time data ingestion, sub-second query speeds, and scalability makes it a perfect choice for businesses that need to analyze vast amounts of time-series and event-driven data. With growing adoption across industries.
Need help transforming your real-time analytics with high-performance querying? Contact our experts today!
Watch the Apache Druid Blog Series
Stay tuned for the upcoming Apache Druid Blog Series:
- Why choose Apache Druid over Vertica
- Why choose Apache Druid over Snowflake
- Why choose Apache Druid over Google Big Query
- Integrating Apache Druid with Apache Superset for Realtime Analytics